PublicationsLearning continuous-time working memory tasks with on-policy neural reinforcement learning
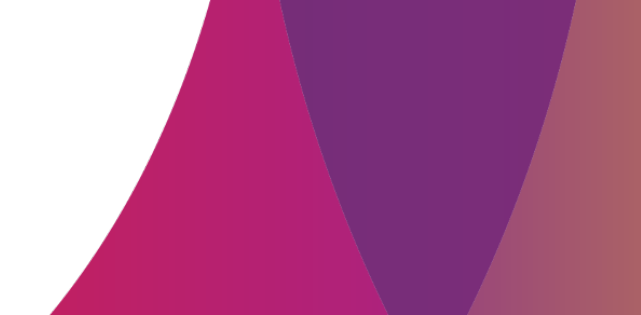
An animals’ ability to learn how to make decisions based on sensory evidence is often well described by Reinforcement Learning (RL) frameworks. These frameworks, however, typically apply to event-based representations and lack the explicit and fine-grained notion of time needed to study psychophysically relevant measures like reaction times and psychometric curves. Here, we develop and use a biologically plausible continuous-time RL scheme of CT-AuGMEnT (Continuous-Time Attention-Gated MEmory Tagging) to study these behavioural quantities. We show how CT-AuGMEnT implements on-policy SARSA learning as a biologically plausible form of reinforcement learning with working memory units using ‘attentional’ feedback. We show that the CT-AuGMEnT model efficiently learns tasks in continuous time and can learn to accumulate relevant evidence through time. This allows the model to link task difficulty to psychophysical measurements such as accuracy and reaction-times. We further show how the implementation of a separate accessory network for feedback allows the model to learn continuously, also in case of significant transmission delays between the network’s feedforward and feedback layers and even when the accessory network is randomly initialized. Our results demonstrate that CT-AuGMEnT represents a fully time-continuous biologically plausible end-to-end RL model for learning to integrate evidence and make decisions.
Support our work!
The Friends Foundation facilitates groundbreaking brain research. You can help us with that.
Support our work